- Publication: Sequoia Capital
- Publication Date: September 22, 2023
- Organizations mentioned: Sequoia Capital, OpenAI, Midjourney, Character.AI, Harvey, Glean, Ava, Pinecone, Langchain,
- Publication Authors: Sequoia Capital, Sonya Huang, Pat Grady
- Technical background required: Medium
- Estimated read time (original text): 20 minutes
- Sentiment score: 70%, somewhat positive (100% being most positive)
TLDR
Generative AI has experienced a rapid rise, with early successes and challenges. As the market enters “Act 2,” companies are focusing on solving real-world problems and creating enduring value for users. Despite current limitations, the shared playbook of model development techniques and emerging product blueprints gives reason for optimism about the future of generative AI.
Methodology:
- Analyzing the market transition from “Act 1” to “Act 2” of Generative AI focusing on customer-centric applications.
- Assessing the performance and revenue generation of startups in the Generative AI space.
- Evaluating the impact of foundational models in the development of novel applications.
Key Findings:
- ChatGPT’s Impact: This model became a significant catalyst in the AI industry, leading to a surge in innovation. It’s growth and adoption were unparalleled, reminiscent of the early internet days.
- AI Research Boom: This period saw AI researchers gaining significant recognition, with a notable increase in AI-related research, publications, and discussions.
- Over $1 billion in revenue generated from startups in the Generative AI realm, which has already had a more successful start than SaaS.
- Generative AI transitioned from technology-driven (“Act 1”) to customer-back approach (“Act 2”) addressing real human problems. The foundational model should play a role as part of the solution, though not the entire solution.
- The Model Development Stack
- Emerging Reasoning Techniques: Developers are enhancing AI models’ complex reasoning abilities with techniques like chain-of-thought and tree-of-thought, narrowing the gap between customer expectations and AI capabilities.
- RLHF and Fine-Tunning: Accessible transfer learning methods such as RLHF and fine-tuning, exemplified by the recent fine-tuning capabilities for GPT-3.5 and Llama-2, allow for the personalization of foundational models to particular domains, enriched by user feedback. Open-source models from Hugging Face are being fine-tuned for superior performance.
- Retrieval-Augmented Generation: Retrieval-augmented generation improves truthfulness and applicability by incorporating business or user context, supported by vector databases from companies like Pinecone.
- New Developer Tools and Application Frameworks: Innovative developer tools (ex: Langsmith and Weights & Bias) and application frameworks are providing reusable components for the creation of sophisticated AI applications and assisting in the evaluation and monitoring of AI models in production.
- AI-first Infrastructure Companies: Coreweave, Lambda Labs, Foundry, Replicate and Modal offer key resources such as scalable GPUs and a PaaS experience, critical for AI development.
- Emerging Product Blueprints
- Generative Interfaces: The landscape of generative interfaces is expanding beyond traditional text-based UX, with innovative user interfaces and authentic-sounding voices from entities like Inflection AI.
- New Editing Experiences: from Copilot to Director’s Mode. Midjourney’s panning commands and Runway’s Director’s Mode, which introduce interactive and intuitive editing controls akin to camera operations, and Eleven Labs allows the user to manipulate voices through prompting.
- Increasingly Sophisticated Agentic Systems. Shifting from rudimentary autocomplete to full-fledged autonomous problem solvers that can handle tasks from start to finish.
- System-Wide Optimization. Autonomously resolving batches of support queries or code requests, which contributes to the overall efficacy of systems.
Recommendations:
- Embrace the customer-back approach to ensure Generative AI applications solve end-to-end human problems.
- Innovators should focus on creating more comprehensive solutions rather than mere novelty applications.
- The competitive advantage in the Generative AI market might hinge more on workflows and user networks than on data moats.
Thinking Critically
Implications:
- A wider adoption of the customer-back approach in Generative AI could lead to more practical and user-centric applications, potentially driving significant economic value. “Act 2”: The market is now transitioning to a phase that emphasizes customer-centric solutions, aiming to solve real-world problems. Companies like Harvey, Glean, Character, and Ava are leading this transition, focusing on genuine user needs.
- If organizations ignore the recommendations, there may be a proliferation of novelty applications with limited real-world utility, possibly hindering the growth of the Generative AI market.
- The discussion around ethics and regulation of machine-generated IP could become a significant political and social discourse, impacting the legal framework surrounding AI.
Alternative Perspectives:
- User Retention: Despite the initial enthusiasm, generative AI faces challenges in keeping users engaged long-term. Data showed that AI-first applications had lower month 1 mobile app retention compared to more established companies.
- Daily Engagement: The median DAU/MAU for generative AI apps stands at 14%, while the median DAU/MAU for imcubents is 51% (Some of the best consumer companies have 60-65% DAU/MAU; WhatsApp’s is 85%), indicating a challenge in getting users to engage with these products daily.
- The rapid growth and adoption of AI led to various challenges:
- Intellectual Property Concerns: With AI generating content, artists and creators began questioning the legitimacy and originality of such content.
- Ethical and Regulatory Debates: The capabilities of AI led to intensified debates over its ethical use, potential biases, and the need for regulations.
- Utility Doubts: As the market became saturated with AI products, concerns arose about their actual utility, especially when some products didn’t live up to the hype.
Glossary
- Cerebral Valley: A term possibly coined to describe a hub or community of AI researchers and enthusiasts.
- Customer-back approach: An approach in Generative AI development focusing on solving end-to-end human problems.
- Data Moats: A competitive advantage obtained through unique data, which might not be as sustainable as believed.
- Foundation Models: Core AI models that serve as a basis for building a variety of applications.

Members also get access to our comprehensive database of AI tools and fundraising
Sequoia Capital
Recommended Research Reports
×
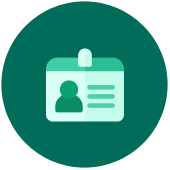
Become a Member to get full access
Subscribe today to unlock the door to a world of unlimited information. Gain full access and never miss out on valuable content again!