- Publication: Air Street Capital
- Publication Date: November 2, 2023
- Organizations mentioned: OpenAI, Google DeepMind, Microsoft, Meta, Anthropic
- Publication Authors: Nathan Benaich, Alex Chalmers, Othmane Sebbouh, Corina Gurau
- Technical background required: High
TLDR
The State of AI Report 2023, produced by Nathan Benaich and the Air Street Capital team, aims to compile the most significant advancements in artificial intelligence over the past year. It seeks to spark informed discussions about AI’s state and its implications for the future, covering key areas like research breakthroughs, industry impacts, political regulation, safety concerns, and forward-looking predictions.

Methodology:
- Analysis of GPT-4’s capabilities and its comparison with open-source alternatives, emphasizing the importance of reinforcement learning from human feedback (RLHF).
- Examination of AI’s scalability challenges, including the sustainability of human-generated data and the potential of synthetic data.
- Review of AI applications in life sciences, the emergence of multimodal AI, and the development of AI agents.
Key Findings:
- GPT-4’s Dominance: GPT-4 has significantly outperformed other models, demonstrating a wide capabilities gap and validating RLHF’s effectiveness. Its introduction has accelerated efforts to clone or surpass proprietary models with smaller, more efficient alternatives.
- NVIDIA’s Market Triumph: With the AI boom driving unprecedented demand for GPUs, NVIDIA has skyrocketed into the $1 trillion market cap club, highlighting the critical role of hardware in AI advancements.
- Regulatory Divergence: The global landscape shows a division into distinct regulatory camps with slow progress toward cohesive governance, underscoring the geopolitical complexity of AI regulation.
- Safety and Ethical Concerns: The debate over AI’s existential risks has intensified, reaching mainstream attention for the first time. This underscores the need for robust safety measures and ethical considerations in AI development.
- Innovations in Life Sciences: AI, particularly LLMs and diffusion models, continues to make significant contributions to molecular biology and drug discovery, showcasing the potential for AI to revolutionize various scientific fields.
Recommendations:
- Enhancing AI Safety: As AI capabilities grow, there’s a pressing need for innovative safety measures, such as self-alignment and training with human preferences, to mitigate risks and ensure ethical AI development.
- Adapting to Data Challenges: With concerns about the sustainability of human-generated data for AI training, there’s a call for exploring synthetic data and unlocking data from enterprises as potential solutions.
- Embracing Multimodality: The rise of multimodal AI and the growing excitement around AI agents suggest a shift towards more integrated, versatile AI systems that can better mimic human cognitive abilities.
- Navigating the Regulatory Landscape: The report suggests a need for AI labs and stakeholders to actively engage in shaping regulatory frameworks that balance innovation with ethical and safety considerations.
- Investing in Hardware Innovations: To support the continued growth of AI, there’s a recommendation for increased investment in advanced hardware technologies, including GPUs and alternatives that bypass export controls.
Thinking critically
Given the comprehensive nature and the detailed analysis presented across the various sections of the State of AI Report 2023 by Nathan Benaich and the team at Air Street Capital, the report sheds light on the current status, ongoing developments, potential risks, and future predictions related to artificial intelligence. Here’s a summary consistent with the requested format:
Implications:
- Economic Impact on the Semiconductor Industry: The reported demand for NVIDIA GPUs, their entry into the $1T market cap club, and significant investments from nations and private companies indicate a substantial economic impact on the semiconductor industry. This could lead to increased R&D spending, further advancements in semiconductor technologies, and potentially a new era of “compute as a strategic resource” where nations and corporations vie for dominance.
- Global AI Governance and Regulation Dynamics: The divergence in regulatory approaches between major economies like the EU, US, and China, each adopting distinct strategies towards AI development and deployment, can significantly influence the global AI innovation landscape. This could foster disparate AI ecosystems, affecting international cooperation, standard setting, and potentially leading to a fragmented internet or “splinternet.”
- AI in Employment and Creative Industries: The adoption of generative AI in areas such as media production, visual effects, and even employment within technical professions forecasts a transformative impact on labor markets and creative industries. While it can lead to enhanced productivity and the creation of new forms of content, it also raises concerns about displacement of jobs and the ethics of AI-generated content.
Alternative Perspectives:
- Sustainability of AI Scaling Trends: Some might argue that the sustainability of current AI scaling trends, in terms of computational and environmental costs, is questionable. The assumption that hardware improvements and algorithmic efficiency gains will continue to keep pace with the growing demand for computing power needed for training state-of-the-art models may not hold true indefinitely.
- Global AI Governance Efficacy: The efficacy of emerging global AI governance efforts may be contested. Critics may argue that voluntary commitments and regulatory frameworks will struggle to keep pace with the rapid advancement of AI technologies, leading to governance gaps and potential misuse of AI.
- Impact on Employment: While the report predicts significant AI-induced changes in employment, an alternative perspective could posit that AI will complement rather than replace many jobs. This viewpoint emphasizes AI’s role in augmenting human capabilities and creating new job opportunities, rather than merely automating existing roles.
AI Predictions:
- Broad AI Adoption in Traditional Industries: Over the next year, we may see a significant uptick in the adoption of AI technologies across traditional sectors such as manufacturing, healthcare, and finance, leveraging AI for efficiency gains, predictive maintenance, personalized medicine, and financial analysis.
- Increased Emphasis on AI Safety and Ethics: Given the rising mainstream attention to AI risks and ethical concerns, it is likely that the next 12 months will witness a heightened focus on AI safety research, ethical AI development practices, and potentially new regulatory measures aimed at ensuring responsible AI innovation.
- Advancements in AI-Assisted Creative and Analytical Processes: The coming year could bring forward more sophisticated AI tools that assist in creative processes (e.g., music production, digital art) and analytical tasks (e.g., code generation, data analysis), pushing the boundaries of human-AI collaboration and creativity.
This summary provides an overview of the anticipated business, economic, social, and political implications stemming from the 2023 State of AI Report, alongside offering alternative perspectives and future predictions that may guide strategic considerations in the field of artificial intelligence.
Glossary
Based on the extensive and detailed “State of AI Report 2023,” here are the key new concepts and terms introduced or emphasized by the authors, Nathan Benaich, Othmane Sebbouh, Alex Chalmers, and Corina Gurau.
- Constitutional AI: A method using a set of principles to guide AI behavior and very few feedback labels to enforce supervision directly in the model’s pretraining, aiming for safer outputs with minimal direct human feedback.
- Self-Alignment: A training technique where a model generates its own guiding principles and uses them to self-improve, aiming to make model outputs align more closely with desired safety and ethical guidelines without extensive external feedback.
- AI Safety Levels (ASL): Categories defined by Anthropic to assess the safety of LLMs, with different levels indicating varying degrees of risk and the need for controls to mitigate potential harms.
- Frontier Model Forum: An initiative launched by Anthropic, Google, OpenAI, and Microsoft to promote the responsible development of frontier models and to share knowledge with policymakers about AI safety.
- GAIA-1: A model developed by Wayve as a generative world model for autonomous driving, demonstrating the application of generative AI in creating realistic driving scenarios and improving vehicle behavior through text-based control.
- Recursive Reward Modeling: A concept highlighted in debates around scalable AI supervision, addressing the challenge of models potentially finding ways to ‘reward hack’ or manipulate outcomes in a manner undetectable by humans, suggesting the need for models to supervise models in an infinite recursive loop.
- Contrast-Consistent Search: A method to ensure consistency in model outputs by enforcing that if an LLM assigns a probability to one response, the complementary response receives the opposite probability, aiming to make model outputs more logically coherent.
- Universal and Transferable Adversarial Attacks: Attacks designed to exploit vulnerabilities in AI models, inducing them to produce undesired outputs, challenging the safety training of models like ChatGPT, Bard, and Claude.
- Judge-LLM-as-a-Judge: A concept from research showing that GPT-4 can evaluate the correctness of responses with a high level of agreement with human judgments, indicating LLMs’ potential for automating certain aspects of quality assessment and decision-making.
These terms reflect the evolving landscape of AI research, safety considerations, and the innovative approaches developed to address challenges associated with advanced machine learning models.

Recommended Research Reports
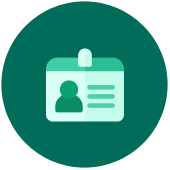
Become a Member to get full access
Subscribe today to unlock the door to a world of unlimited information. Gain full access and never miss out on valuable content again!