- Publication: CXO Talk
- Publication Date: February 28, 2023
- Organizations mentioned: EY, Google, Microsoft, IBM
- Publication Authors: CXOTalk
- Technical background required: High
- Estimated read time (original text): 90 minutes
- Sentiment score: 75%, somewhat positive
Big Ideas
Generative AI Creates Endless Opportunities Generative AI like ChatGPT has endless possibilities for enterprises to systematize knowledge, provide better advice to clients, and act as an “intelligent agent” supporting humans. However, these tools are still early in development.
Responsible AI Requires Governance There is a need for governance structures and editorial oversight to manage organizational knowledge and prevent issues around bias, ethics, and misinformation. Humans must remain accountable.
Knowledge Management Will Be Transformed By codifying institutional knowledge, generative AI can make expertise more accessible. This could help with training, free up time for higher-value work, and increase job satisfaction.
Expect Exponential Innovation Major tech companies are racing to develop better generative AI, so expect extremely rapid iterations and innovations in capabilities. Enterprises cannot afford to lag as followers.
Avoid Overhype and Disappointment While the potential is enormous, these tools cannot match human abilities yet. Overinflated expectations often lead to failures of adoption.
More Transparency Needed Future iterations of generative AI need more transparency around training data and processes to monitor for issues like bias. This will be key for establishing trust.
Thinking Critically
Implications
- Adoption of generative AI within enterprises could significantly enhance efficiency and innovation, leading to the development of new products and services. This could result in a competitive advantage for early adopters and potentially disrupt existing markets and industries.
- If organizations fail to adopt generative AI, they may fall behind in terms of operational efficiency and miss out on leveraging data for strategic decision-making, potentially leading to a decline in market share and relevance.
- The widespread implementation of generative AI could have substantial workforce implications, necessitating reskilling of employees and possibly leading to job displacement in roles that become automated. It also raises ethical considerations around the use of AI, including bias, privacy, and accountability.
Alternative Perspectives
- The speakers may not account for the potential resistance from employees and customers who are wary of AI’s impact on privacy, job security, and the quality of human interaction.
- There might be an overestimation of the current capabilities of generative AI, and some organizations could find that the technology does not yet meet their specific needs or integrate seamlessly with their existing systems.
- The conversation could underestimate the complexity of implementing AI at scale, including the challenges of data governance, infrastructure requirements, and the need for continuous monitoring and updating of AI models.
AI Predictions
- In the near future, we may see a surge in strategic partnerships between AI technology providers and enterprises, aiming to co-develop bespoke AI solutions that cater to specific industry needs.
- There is likely to be an increase in the demand for professionals with expertise in AI ethics, as organizations seek to navigate the social and legal implications of deploying generative AI technologies.
- Predictive analytics powered by generative AI will become more sophisticated, leading to more accurate forecasting in sectors like finance, supply chain management, and healthcare, thereby enabling better decision-making.
Glossary
- Generative AI: AI that can generate new content or data that is similar to but not identical to data it has been trained on, often used for tasks like image creation, natural language processing, and more.
- Deep Learning: A subset of machine learning involving neural networks with multiple layers that can learn progressively higher-level features of the data.
- Machine Learning: A field of artificial intelligence that uses statistical techniques to give computers the ability to “learn” from data, without being explicitly programmed.
- Natural Language Processing (NLP): A branch of AI that focuses on the interaction between computers and humans through natural language, enabling computers to understand, interpret, and generate human language.
- AI Ethics: The field of study that considers the moral implications and societal impacts of artificial intelligence, including issues of bias, fairness, transparency, and accountability.
- Data Privacy: The aspect of data protection that deals with the proper handling of data concerning consent, notice, and regulatory obligations.
- Algorithmic Bias: Systematic and repeatable errors in a computer system that create unfair outcomes, such as privileging one arbitrary group of users over others.
- AI Governance: The strategies and policies for managing and overseeing AI systems within an organization, ensuring they are used responsibly and ethically.
- Digital Transformation: The integration of digital technology into all areas of a business, fundamentally changing how the business operates and delivers value to customers.
- Scalability: The capability of a system, network, or process to handle a growing amount of work, or its potential to be enlarged to accommodate that growth.
- Innovation Ecosystem: A network or environment that facilitates the interconnection of people, organizations, policies, and resources to promote innovation within a particular field, such as AI in an enterprise setting.

Recommended Research Reports
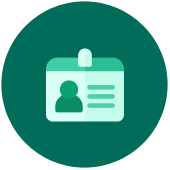
Become a Member to get full access
Subscribe today to unlock the door to a world of unlimited information. Gain full access and never miss out on valuable content again!